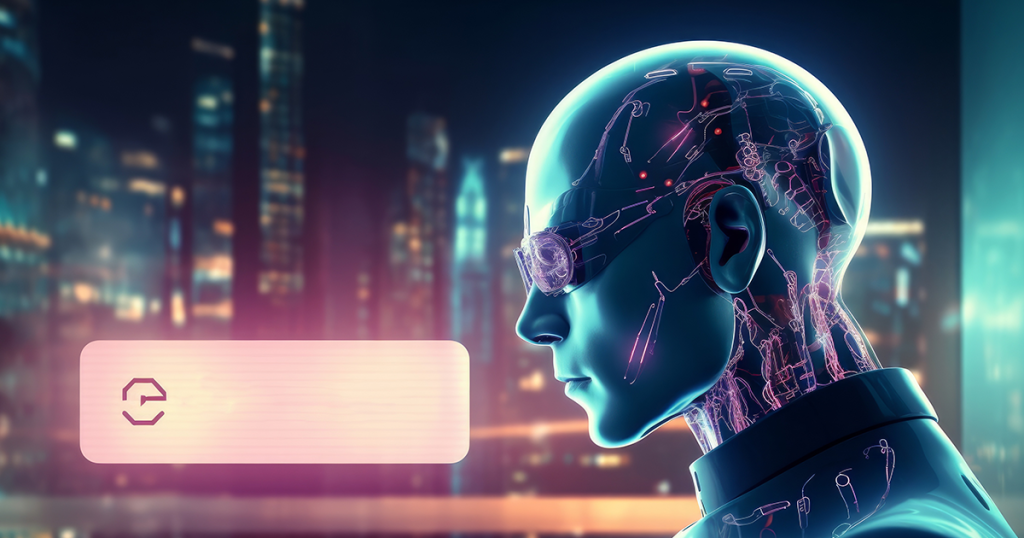
Autonomous advancement of spoken dialogue systems through gradual knowledge acquisition during dialogues
It is one of the intellectual functions of human beings to learn new knowledge through talking with others. In particular, there are a lot of knowledge that can be learned by talking to a person in the situation, not just about general facts. Current dialogue systems use knowledge that was learned from prior data or manually constructed in advance. Our goal is to create “a system that gets smarter as we talk to it.”
Multimodal dialogue system that can read the unspoken information of the dialogue partner
Humans understand not only the literal meaning of the words spoken by the dialogue partner, but also his/her atmosphere and the way he/she speaks. That is, to understand the dialogue, the system needs to take into account not only the verbal content, but also facial expressions, tone of voice, and so on. We are developing technology to estimate how a human interlocutor is feeling by making full use of visual information, audio information, and even physiological signals such as electroencephalogram (EEG) and skin conductance.
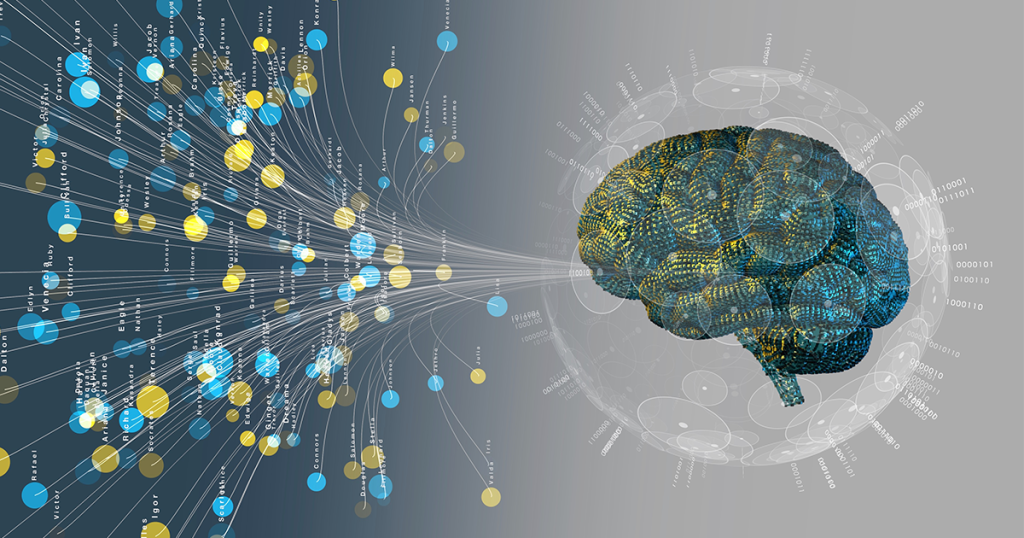
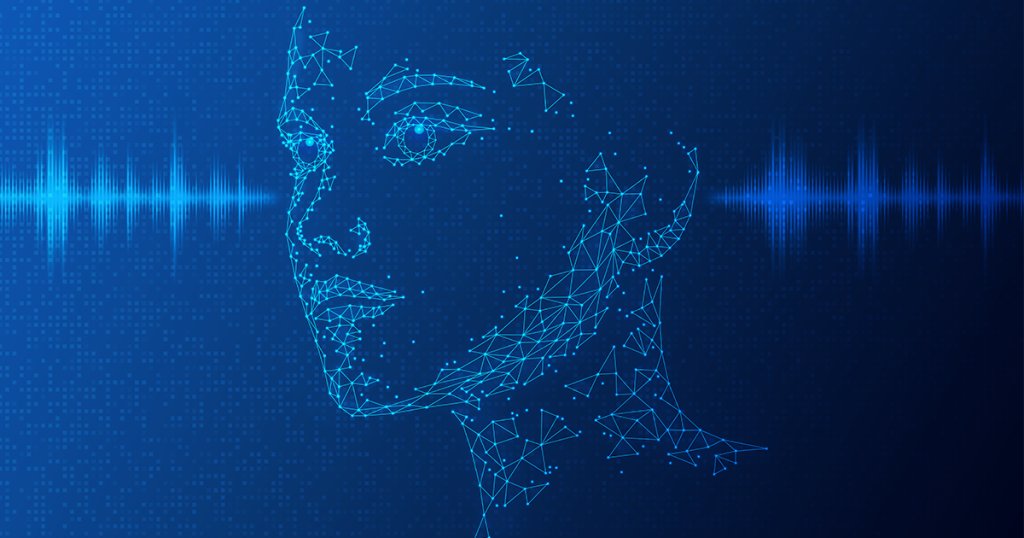
Spoken dialogue processing to address errors and breakdowns at several layers
Communication using spoken language requires that the actions of two interlocutors need to be joint actions at each of channel, signal, intent, and dialogue layers, as well as the communication between systems. That is, errors and breakdowns are caused by discrepancies in some of these layers. Since errors are inevitable when using speech and language, we aim for a spoken dialogue system that is robust against errors by identifying and addressing the causes of such discrepancies.
Automatic model adaptation to users based on unified modeling of spoken dialogue system
We are developing a function that allows spoken dialogue systems to “be adapted” to the user through conversation. For example, the system will gradually become able to recognize the user’s speech and understand the user’s words and meanings without any discrepancies. This corresponds to adaptation of the system’s internal models, such as speech recognition, to the user through conversation. We aim to achieve such functions by using pattern recognition and machine learning techniques based on a unified modeling of spoken dialogue system.
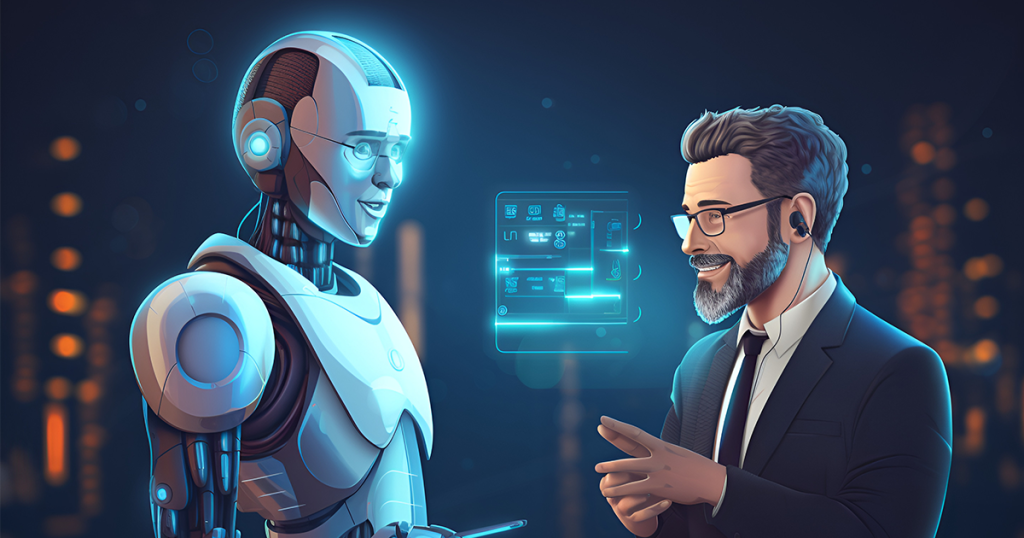
Introduction Videos
built by a student at my previous school.
(in Japanese)
(in Japanese)
YUME NAVI Articles
- Create robots that can talk naturally with humans (in Japanese)
- How to make a robot smarter? (in Japanese)
Press articles
2020.10
ResOU – Hazumi datasets for dialogue systems that recognize human sentiment released
The Asahi Shimbun (in Japanese)
EurekAlert! – Hazumi datasets for dialogue systems that recognize human sentiment released
2017.12
ResOU – Technique to allow AI to learn words in the flow of dialogue developed
THE SANKEI SHIMBUN (in Japanese)
EurekAlert! – Technique to allow AI to learn words in the flow of dialogue developed